Paving the Road for Machine Intelligence
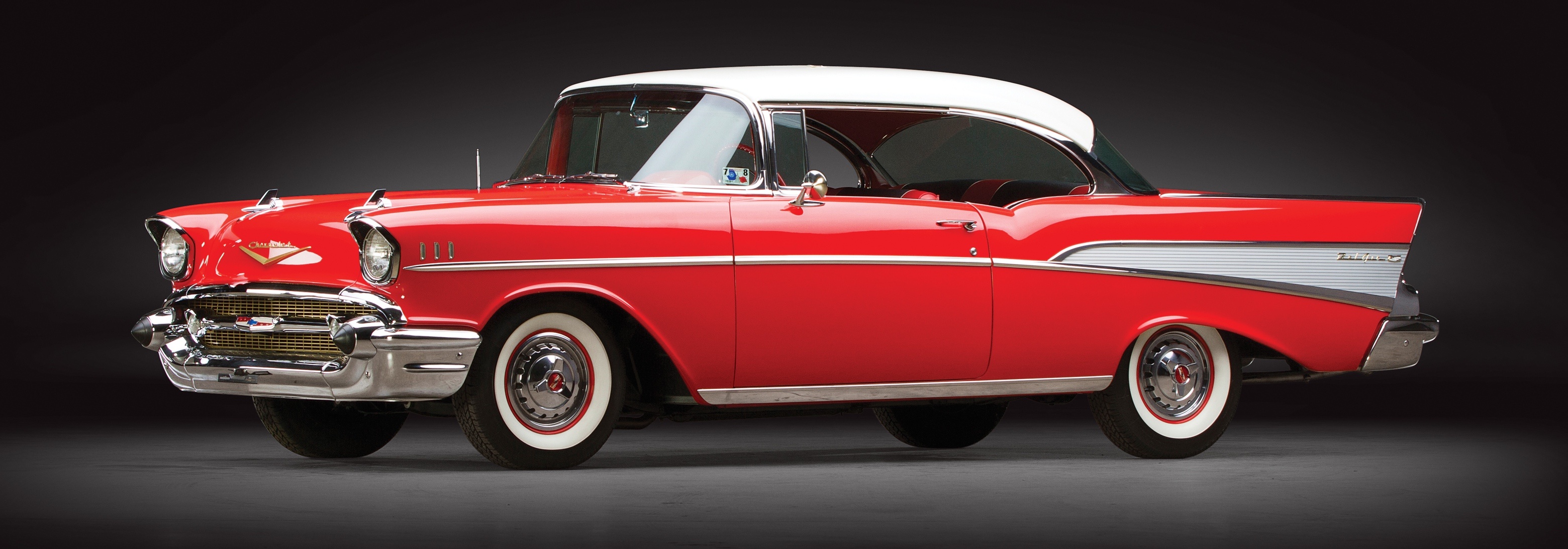
57' Chevy [Photo credit: www.ClassicCars.com]
Welcome to Visage Imaging’s enhanced blog! We’ll be sharing insights about Enterprise Imaging that we hope you’ll find both informative and useful.
Earlier this year, Visage had the opportunity to attend SIIM’s Conference on Machine in Intelligence in Medical Imaging, held in Alexandria, Virginia, Sept 12-13, 2016. SIIM invited attendees to blog about topics inspired by the meeting, and posted the below blog from Brad Levin, General Manager, North America, Visage Imaging, on the new eMIMI Online Group.
Chevy, Tesla and Visions of the Road Ahead for Machine Learning in Medical Imaging Integration
Link to the SIIM eMIMI Online Group Blog
The takeaways from SIIM’s Conference on Machine Intelligence in Medical Imaging (C-MIMI) are both lasting and visual. On the one hand, an extensive list of luminary speakers and researchers painted a vibrant picture of the road ahead, filled with visions of staggeringly accurate, augmented radiologist interpretation and impressive convolutional neural networks that spotlight disease only today’s most expert, sub-specialty trained radiologists can pinpoint. The vision of machine intelligence powered by magical GPUs is undisputedly impressive, and it does appear we’re on the cusp of making a difference in so many ways.
On the other hand, we’re at a clear fork in the road. One of the systemic hurdles Imaging faces is the vast majority of the industry is ‘driving’ on top of an informatics infrastructure based on decades old “structured, stoic systems” wrought with significant limitations. Dr. Dreyer, C-MIMI’s Keynote Speaker, openly posed the question in his address The Trajectory for Machine Learning in Medical Imaging, “Are our current systems capable to support these innovations?” After discussing the vision and investment behind MGH’s Clinical Data Science Center, Dr. Dreyer commented about the challenges of machine learning integration with an autonomous car analogy. He explained that a Tesla is just ideal “waiting to be automated” with a digital dashboard, power this and that, and doesn’t use gas. “Imagine trying to automate a 55’ Chevy?” he continued. Dr. Dreyer went on to explain, “Now, I’m not saying our [Partners Healthcare’s] EHR, which we spent $1.5B on is a 55’ Chevy, but maybe it’s a 57’ Chevy!”
Developed way back in 1966 and ironically also at MGH, the underbelly of today’s most widely installed EHR is based on MUMPS (Massachusetts General Hospital Utility Multi-Programming System). Similarly, the PACS used at so many academic, inpatient and outpatient institutions was developed last century, based on dated technology ill equipped for today’s Imaging practice, let alone a future infused with machine intelligence. I vividly recall attending SCAR/SIIM meetings in the early days of PACS, where speaker after speaker voiced the caution, “Imaging datasets are only getting bigger.” Back then images were sized in MBs, but how prophetic is that statement now, with today’s largest current/prior studies measured in tens of Gigabytes? It struck me that Imaging is yet again at a technology crossroads. Consider the need to display massively large studies, integrated with the results of machine intelligence algorithms mining vast repositories of images, all presented to the reading radiologist in a non-disruptive interpretation experience and you’ve got a nearly impossible challenge working within the bounds of archaic infrastructure. If not now, when? Is there any time better than the present for the Industry to finally turn the corner on last century’s PACS? GPUs are powering an Imaging revolution, don’t get left behind.
RSNA 2016 is almost here! Please contact us to schedule a priority demonstration.