Imaging AI in Practice | Is It Real Yet?
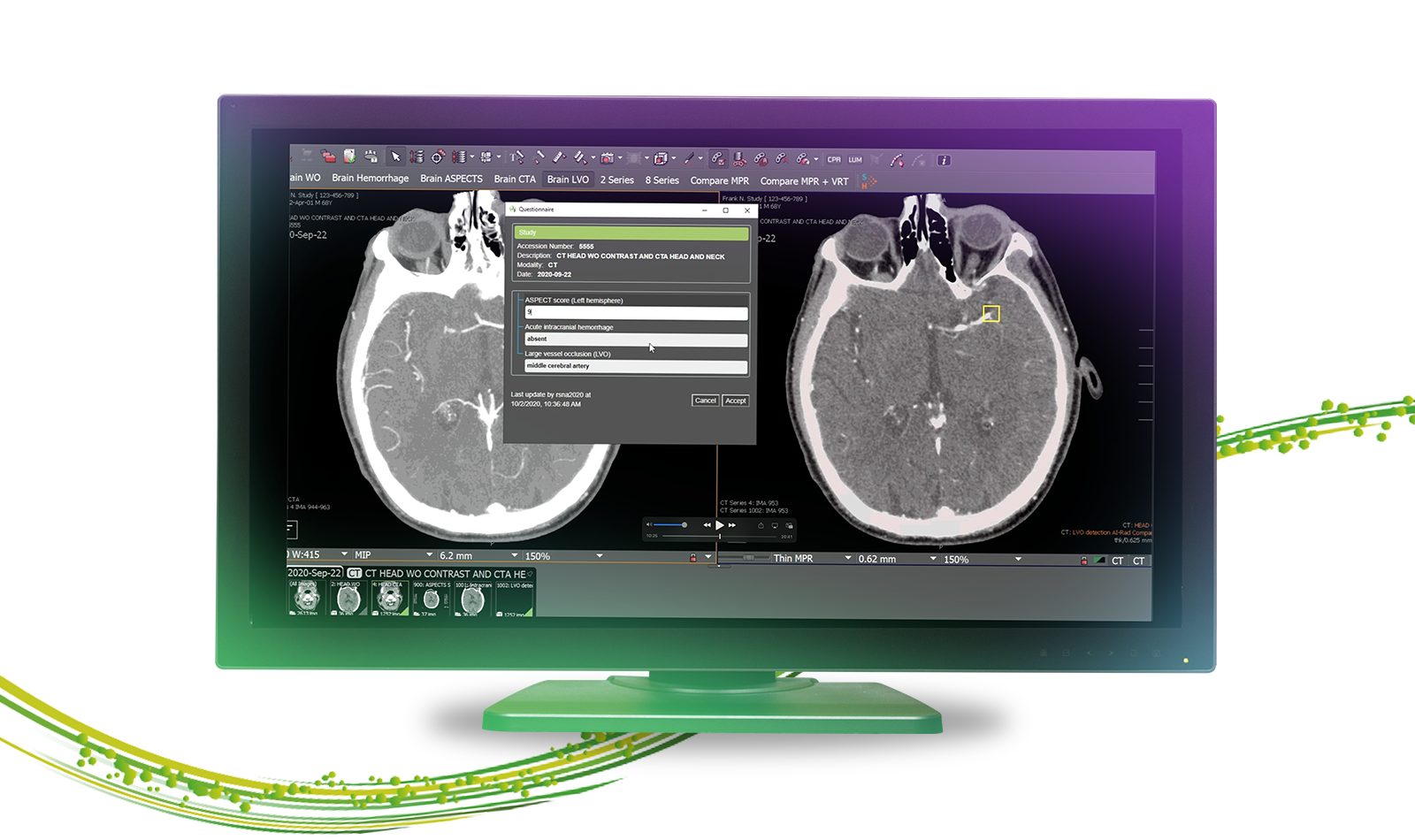
There is a strong belief in radiology that Imaging AI is “coming soon” and promises to improve efficiency and accuracy of radiology operations, and ultimately, patient outcomes.
Over the last few years in the medical imaging industry, companies all around the world, both large and small, jumped on the AI bandwagon, partnering with leading healthcare institutions in the quest for the panacea of AI powered apps. Many healthcare institutions themselves have been investing significant resources in the same domain. However, most research endeavors, if they are successful, only end in a publication and do not translate into actual clinical use. And those that do advance to obtain regulatory clearance and implementation into routine clinical use may only be useful and valuable for the institution that created the algorithm/app.
Generalizability, transferability and out-of-the-box (turnkey) implementation at other institutions is a common hurdle. One of the primary reasons is because the workflow architecture is unique or custom-built for every institution. Specifically, there is significant variability in how different applications and informatics systems interact at different institutions. This also includes the degree of standardization in the mechanism for data exchange, data formats and interpretation of the data flowing between applications.
In an effort to increase interoperability, the Integrating the Healthcare Enterprise (IHE) radiology initiative started more than 20 years ago with the goal to standardize integration mechanisms in radiology departments. This effort has grown to encompass multiple domains and dozens of Integration Profiles: the unambiguous, detailed specifications of different workflows that allow users of the healthcare IT systems to derive maximum value from the systems that integrate easily and fit into the specific environments of different healthcare organizations. Visage is a corporate IHE member, and I represent Visage on the IHE Radiology Technical Committee.
In 2020, the IHE Radiology domain developed and published for trial implementation, two integration profiles aiming to codify and standardize the aspects of incorporating AI models and applications into the workflow of radiology practices:
- AI Results
The AI Results profile specifies the data formats of the results after running the AI algorithms on imaging datasets. For example, this may be the simple identification of the presence or absence of particular feature, classification of a lesion, set of measurements or a segmentation of an image region of interest.
- AI Workflow for Imaging
The AI Workflow for Imaging profile provides the definitions of controlling the AI model invocation and data exchange that supplies source data to the models and feeds the results into other applications and systems.
The Imaging AI in Practice (IAIP) Demonstration planned for the upcoming RSNA 2020 brings together vendors whose products encompass the radiology workflow, from the time of ordering a patient’s study to the acquisition of images and interpretation by the radiologist – with AI models and algorithms utilized at each step of the workflow. The IAIP demonstration will be spotlighted in the AI Showcase at RSNA 2020 to see how AI could be integrated into your daily clinical radiology practice. Visage is a proud participant in the IAIP, a multi-vendor interoperability demonstration showcasing new technologies and communications standards needed to integrate AI into the diagnostic radiology workflow. Using real-world clinical scenarios, 16 vendors demonstrate more than 26 systems, such as PACS, reporting and AI applications, to show how AI can be used to support improvements in patient care.
An example used in the IAIP demonstration, Visage 7 Workflow can reprioritize the interpretation worklist based on the information received from the AI model that identifies a patient with a stroke condition, and then presents the results of the AI analysis and classification as an integral part of the Visage 7 In-viewer Workflow, all while the radiologist is interpreting the current study. When you view the IAIP demonstration, keep in mind that all of the AI interoperability capabilities shown with Visage 7 are available today.
Another area where there is significant value for standards-based data exchange is in the area of research and development for new AI models, including: to accelerate data labelling and annotation for training, to validate and test AI algorithms and to obtain user feedback. Visage has the expertise with our Visage AI Accelerator solution to orchestrate the complete AI pipeline using Visage 7 as the foundation for ground truth curation through the embedding of multiple different AI models directly into interpretation workflow. One example is our annotation capabilities using FHIR-based webforms that leverage LOINC coding. In other words, annotations are well organized and searchable, and the webform can include additional annotations, including patient characteristics and imaging feature descriptions, along with free text. Visage also supports Semantic Annotations to further streamline the annotation process and create rich, mineable data. Suffice to say, Visage’s capabilities are feature-rich and flexible, and enable accelerated translation from code to bedside.
Visage has always been a proud supporter of well-defined and practical standards that improve interoperability in medical imaging. Visage is active and will continue to participate in the definition of next generation standards supporting all phases of research, development and deployment of modern AI algorithms and applications. Please be sure to make time to see the IAIP demonstration at RSNA 2020 and let me know what you think.
To learn more about Visage's exciting activities at RSNA 2020, and to schedule a Priority Demonstration, please click here.
[Note: A big thank you to MingDe Lin, PhD, Visage’s Clinical Research Director, North America, for collaborating with me on this blog post!]